Taking the First Step Towards NDE 4.0
Part 6: Archiving and Meta-Analysis of NDE Data
In this sixth article on the topic of NDE Automation, we will discuss archiving of NDE data, the meta-analysis of that data, and its ultimate usefulness in achieving operational efficiencies amongst other product and process improvements.
Why Archive Our NDE Data?
It is often a regulatory or a commercial requirement that NDE records of inspection must be maintained for the expected life of the item, or for a specified number of years from the date of inspection. Some requirements for record keeping are now dictating that the raw data be retained, not just the final acceptance report, or a summary of the findings.
However, there are many benefits to be gained from archiving the NDE data, beyond compliance with regulatory requirements or client expectations. As we discussed in a previous post “Data is the new oil.” Acquiring NDE data requires investment of capital and ongoing operating costs. It makes commercial sense therefore, to extract as much value as possible from those investments.
The certification of an inspected item as being fit for service is the primary purpose of the NDE process. The maintenance of inspection records as proof of this suitability is the primary purpose of archiving the inspection results. These goals need not be the only use of the data used to arrive at that certification of fitness.
Proof of an item’s suitability is the purpose of any individual NDE data file. As a group, however, NDE files become a library of useful information that extends their value beyond the individual item. The aggregated data has value to a surprisingly wide audience. One obvious use for this library of NDE data is meta-analysis.
What is Meta-Analysis?
Meta-analysis is a statistical study performed on a group of data sets that have related content. Most automated NDE processes are collecting and analyzing on an item-by-item basis. Meta analysis looks at the aggregated data from many inspections.
Anyone who has worked with a process capability or POD (Probability Of Detection) curve will be familiar with one of the more common examples of meta-analysis of NDE data. Data from the inspections of a large number of specimens is used to define the capability an inspection method to reliably detect flaws of a desired minimum size.
In a manufacturing context, the inspection data files naturally accumulate over time, to provide a library of information that can be mined for insights about the products and the processes that made them. In the context of fixed assets like oil and gas infrastructure, or power generation equipment, there maybe a small collection of files from periodic in service inspections of that one component over its lifetime. These files can be aggregated with data files from inspection of similar components in the same facility - or indeed from inspections performed at many facilities worldwide – to create a much deeper data library for meta-analysis.
How is Meta-Analysis Useful?
Meta analysis provides a broad overview and specific insights about a manufacturing process and the products that it produces. The metaphor that “You cannot see the forest for the trees“ perfectly describes an individual instance of any NDE process: the inspection of an individual item seeks to find defects that are often microscopic in scale.
Taken on an individual basis, no single NDE data file can give insight about an item’s performance over time, or its characteristics relative to others of the same type. Each NDE data file describes only a single item, while a library of such data files can be analyzed in the aggregate to produce insights about the product, the manufacturing process, and even the NDE process itself.
The variety of questions that can be asked is almost limitless. A few of the more obvious questions include:
- Has the product quality changed over time?
- Does one production line have higher quality than another?
- Are some defect types more common that others?
- Do specific defects occur more often on specific production lines?
- How can we improve the analysis process - how do we obtain better detection and classification of indications within the NDE data? Can we increase the probability of detection (POD) ?
- Is there other valuable information within the data file that we are not currently using for the Accept / Reject decision
Questions 5 and 6 are both about seeking improvements to the NDE process and are what drives much of the interest in machine learning (ML) or artificial intelligence (AI) within the NDE industry.
Question 6 is of particular interest to manufacturers and owners of long-lived assets like oil and gas infrastructure, aircraft and their engines, and electrical power generation equipment. There are many examples within aerospace and power generation NDE where historical data has been used to improve lifespan predictions, as failures and destructive testing prompts re-examination of data archives.
Must We Archive All of the Raw Data?
No.
If storage space were infinite and costs to maintain the data storage media were not considerations, we might be happy to store all of the raw data, forever. However, this is usually impractical. Without a purpose and a plan, data will accumulate like memorabilia in our grandparent’s attic. There may indeed be useful things stored there, even treasure, but much of it is of no practical use, and will be forgotten over time. We need to plan what will be archived and why.
For example, the study for improvement of a manufacturing process can be achieved by archiving and analyzing of the summary data form product inspections: quality trends easily emerge from statistically studying how many defective parts occur across various product lines, suppliers and manufacturing facilities. This type of summary data is relatively compact and is therefore easy to archive.
More in-depth analysis requires more in-depth data to study. For example, modern image and data analysis may be able to reveal “proto-flaws” that may once have been classified as benign variations when the original inspection was conducted decades ago, but might now be able to predict a limitation upon a component’s suitability for refurbishment and life extension. The answer to the “Why?” question will tell us what we need to archive.
The Role of Automation in Data Archiving
Archiving is a natural extension of the automated analysis and reporting steps. Automation can make the process seamless and even provide multiple archives with subsets or summaries of inspection data, to suit particular audiences and purposes.
Automation is also important for the use of the archive itself. Purpose built software and database macros can easily be programmed to crawl through large libraries of archived data, extracting files with particular characteristics, or summarizing trends within those files. This curation is an essential step in any meta-analysis, or preparation of training data sets for use in machine learning.
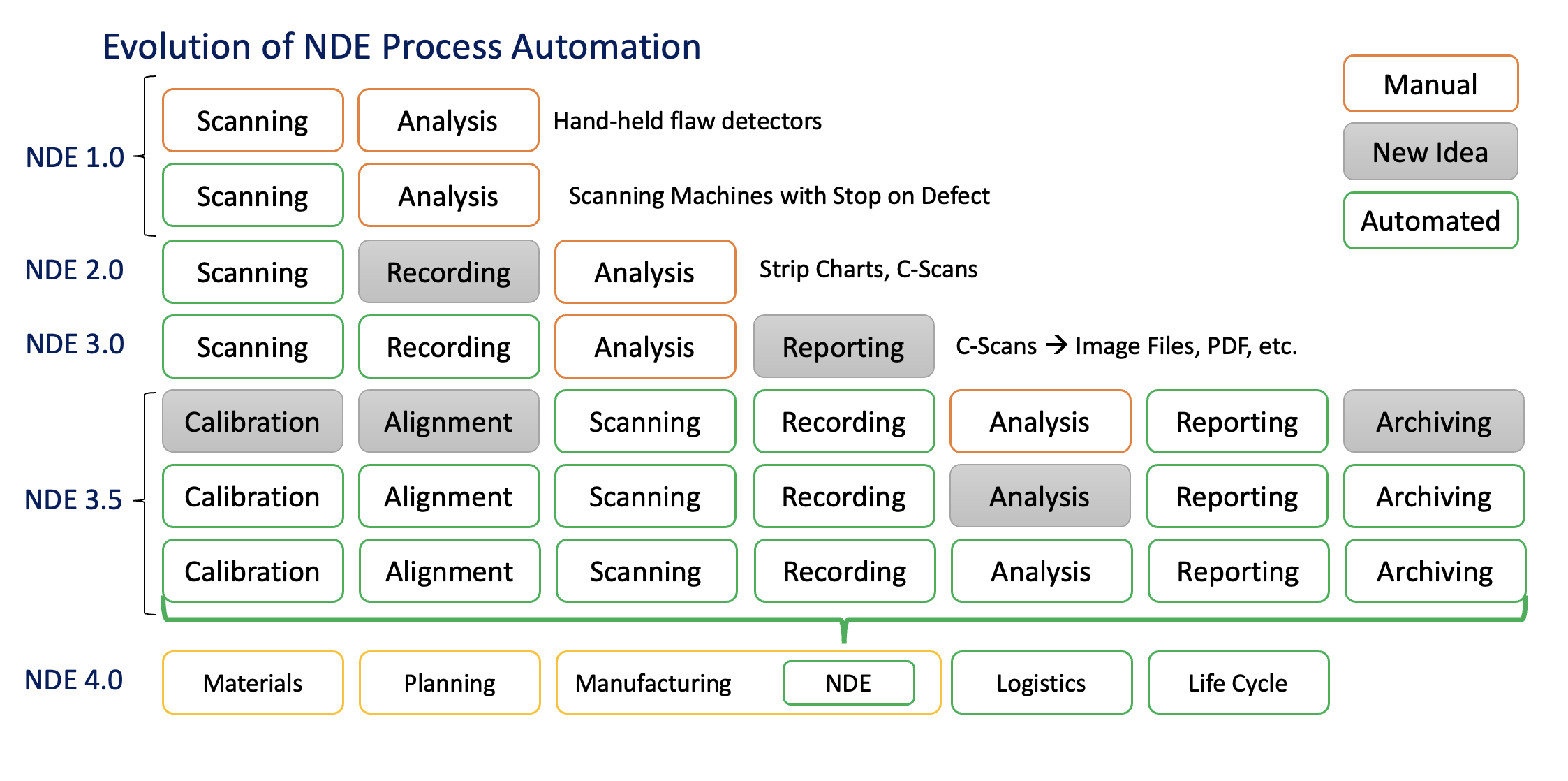
Image adapted from: W. Weber, D. Mair, D. Jansen, L. Lombardi , ’Advances in Inspection Automation’
QNDE Proceedings, 2009
Stay Tuned. Our last post in this series will explore how this all fits together.

UTEX is attending the ASNT Research Symposium this week. Want to chat about NDE 3.5, our instruments, or your NDE challenges, reach out to us set up a meeting.
More in "Taking the First Step Towards NDE 4.0" series
|